AI has shot into prominence in the past year. Market leaders like Google and Microsoft are already introducing AI-backed products and the rest of the world is following suit.
AI has become so relevant that bias has come into play regarding AI solutions.
But why should we even account for AI bias, and does it exist right now? The easy answer is yes! DataRobot surveyed technology leaders in 2022, and they found that 56% of them fear AI bias will lead to loss of customer trust.
In this article, we’ll dig into what AI bias means, different types of AI bias, and real-life examples. Alongside, we’ll explore what to do about AI bias and how to make it less biased as you plan your business strategies for 2024.
So, let’s dive in and explore this important topic together!
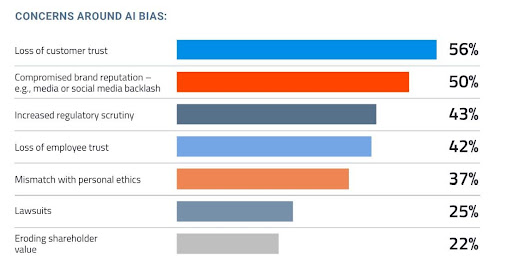
Image sourced from datarobot.com
What is AI Bias?
AI bias has been called different names—algorithm bias and machine learning bias to list just two. Artificial intelligence bias happens when there are unfair or systematic discrepancies in the AI systems that predict or make decisions.
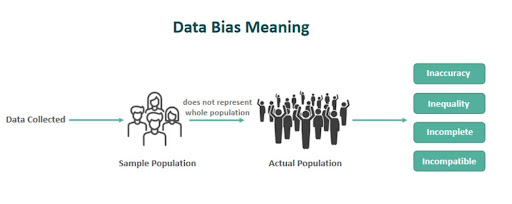
Image sourced from wallstreetmojo.com
While artificial intelligence biases or prejudices can emanate from different sources, the results are the same: they produce results that are discriminatory or unfair towards certain groups or individuals based on several factors such as age, gender, race, and socioeconomic status.
Often, AI biases happen when programmers make assumptions during the algorithm development process or train the algorithm with biased data.
Types and Examples of AI Bias
Below are the most common examples of what AI bias can look like in 2024:
Training Data Bias
This bias arises when the input data used to train an artificial intelligence system doesn’t fully represent the population it serves. When there is training data bias, the AI will make biased decisions and recommendations that will negatively affect some groups of people. Training data bias is a common AI chatbot mistake and is also prevalent with decision-making AI tools.
A good example was an incident that happened in 2019. There was an algorithm used in many United States hospitals to determine which patients required further medical care.
Some researchers found that the algorithm favored white patients over their black counterparts by a large margin. This algorithm used datasets of patients’ previous healthcare expenses for training purposes. Although the algorithm itself didn’t use race in its decision-making, black patients have historically incurred lower costs than white patients with the same conditions.
For this reason, the algorithm gave white patients higher health risk scores, meaning that they were more likely to be chosen by the hospitals for extra treatment programs like nursing appointments than black people with the same illnesses.
Algorithmic Bias
Algorithmic bias is another common type of AI bias, and it happens when the bias comes from the AI’s design or implementation. Algorithms can produce bias on account of their design or certain features they have come to recognize over time. This type of biased algorithm can also unintentionally favor or disfavor a group or groups of people.
For example, say a company receives over a thousand job applicants for a role they intend to fill within the next month. To get the job done faster, they employ the service of an AI or ATS (Applicant Tracking System). This ATS has previously been trained on hiring data and, therefore, looks for patterns in the resumes of the candidates.
If previous data suggested that people submitting applications via the organization’s US or Canada Only Domains website, the ATS can begin to favor them in this new data even if they are not the most qualified. This means the ATS will screen out candidates from other countries even if they are qualified for the role.
User Bias
You have a user bias when there is an issue with input data. User bias can happen when users intentionally or unintentionally enter false or discriminatory data that strengthens bias already present in the system.
Imagine inbound call center technology using AI to make the staff’s accents sound American when talking to United States customers. This helps make communication smoother and reduces mistakes. But here’s the catch: they only use this voice-changing tech for American customers.
The issue is that the AI assumes only Americans would like or need this voice change. This is a bias—it’s unfair because it doesn’t think about customers from other countries who would enjoy an improved customer experience with an American accent in the mix.
Technical Bias
A situation can arise where the software or hardware used to deploy or develop an AI system introduces bias into the system. This is a technical bias.
The programming teams may be constrained or lack resources like storage capacity or computing power. In that event, all AI and machine learning solutions will be trained on a limited dataset. In such a situation, the algorithm will be less accurate or give biased results due to inadequate exposure to a more diverse data set.
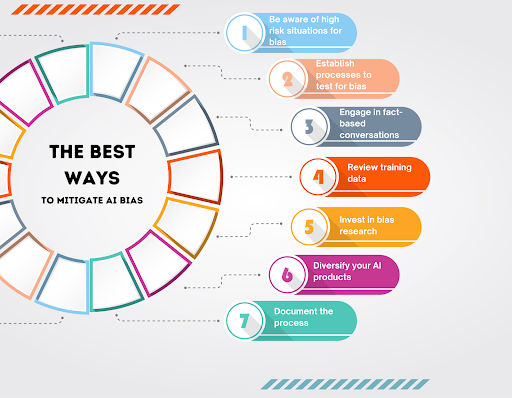
Steps to Mitigate AI Bias in 2024
To mitigate bias in the machine learning algorithm, consider all the endpoints through which bias could be introduced into the system. The endpoints will determine which techniques to leverage for a bias-free AI system.
With that in mind, the following techniques will help you avoid and account for bias while deploying AI solutions:
Pre-processing techniques
As the name suggests, pre-processing techniques involve changing the input data before it is fed into the algorithm. Doing this will create a more representative and diverse dataset, which will help mitigate AI bias and inform on what to do about AI bias.
Some examples of pre-processing techniques that help on how to avoid AI bias include the following:
- Balancing and sampling: Here, you want to ensure the dataset considers all applicable user groups. Use methods like undersampling and oversampling to accomplish this. Balancing and sampling avoid bias and enhance model accuracy when done correctly.
- Data augmentation: With data augmentation, you’re concerned with generating new data points to increase the representation of underrepresented groups in the dataset. For example, if the input data contains limited samples of a certain group, data augmentation will be used to increase this group’s size and the entire dataset’s diversity.
Algorithmic techniques
The second technique to mitigate AI bias for business communication tools in 2024 is adjusting the algorithm. Techniques to do that are known as algorithmic techniques, and they include the following:
- Regularization: Regularization addresses overfitting in training data by introducing a penalty term into the algorithm’s loss function. This strategy reduces bias and improves the model’s predictive accuracy when used in real-world scenarios.
- Adversarial Training: In the realm of model training, an advanced technique involves the deliberate exposure of the model to adversarial examples to help solve bias in machine learning algorithms. The examples—read as “challenges”—are designed to trick machine learning models. You’re improving on the model’s capabilities by challenging the algorithm with unique examples that don’t follow standard training models. As such, it becomes better at recognizing and refining its output to fit variations in input data.
- Fairness Constraints: In the dynamic landscape of bias in machine learning algorithms, a sophisticated approach involves the strategic imposition of constraints during the model’s optimization process. By integrating constraints like demographic factors and accounting for protected groups while training the model, you can generate outputs that are fair across different demographic groups.
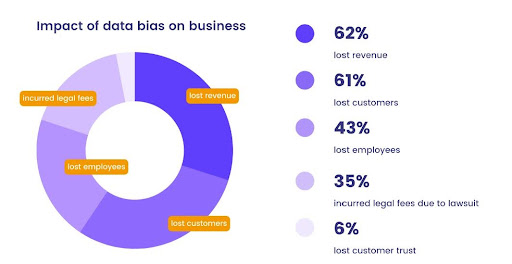
Image sourced from statice.ai
Post-processing techniques
Post-processing techniques strive to identify and eliminate bias in virtual machines and their learning algorithms by scrutinizing their outputs after the training phase.
Some examples include the following:
Bias Metrics
This approach involves measuring the level of bias present in the model’s predictions, utilizing quantitative metrics such as equalized odds and equal opportunity. These metrics play a crucial role as effective tools in both identifying and correcting inherent biases within the model.
Consider a business that uses a credit scoring model to assess loan applications. If the model favors certain demographic groups over others, it may result in biased outcomes. In this context, you can deploy bias metrics to assess whether the approval rates are consistent across different demographic categories.
If the equalized odds metric indicates disparities, it signals the presence of bias, prompting developers to reevaluate and adjust the model to ensure fair and unbiased lending decisions for all applicants. In recent times, orchestration tools have been used in this area.
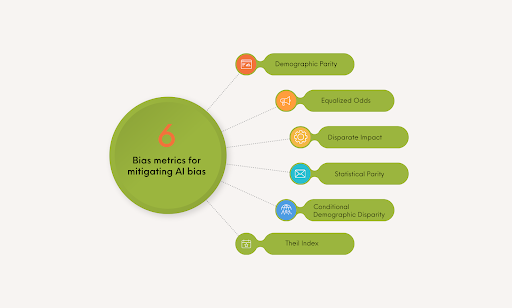
Explainability
This is about making the model’s predictions easy to understand. It does this by explaining, like pointing out the important things that helped the model decide. Explainability helps clarify how the model works and makes it more responsible for its results.
Fairness Testing
This is testing to see if the model is fair. You can use different tests, like looking at statistical parity and checking for disparate impact or individual fairness. Fairness testing helps find any unfairness you may have missed when training the model.
Remove AI Bias by Testing the System Before & After Deployment
In the long run, ensuring AI plays fair is crucial so that the AI systems we create work well and people can trust them. To do this, you must understand AI biases and know how the different types of machine learning models can affect output data as described above.
Examining AI trends in 2024, it is clear that we’ve got a chance to use smart plans to reduce bias. This means using different and fair training data, creating algorithms that play fair, and always keeping a close eye on developments. If you make fairness a big deal in AI, you can integrate technology that provides a fair, equal experience for all visitors to your online business.
- The Rise of Intelligent Websites - February 19, 2025
- Top Trending Products to Boost Your Shopify Store in 2024 - September 4, 2024
- AI Terms Glossary: Key AI Concepts You Should Know - August 22, 2024